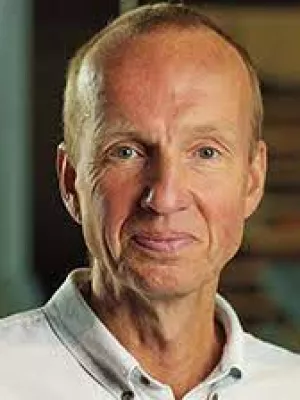
Petter Pilesjö
Professor
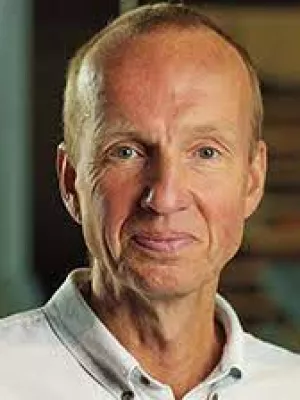
Understanding the spatial non-stationarity in the relationships between malaria incidence and environmental risk factors using Geographically Weighted Random Forest : A case study in Rwanda
Författare
Summary, in English
As found in the health studies literature, the levels of climate association between epidemiological diseases have been found to vary across regions. Therefore, it seems reasonable to allow for the possibility that relationships might vary spatially within regions. We implemented the geographically weighted random forest (GWRF) machine learning method to analyze ecological disease patterns caused by spatially non-stationary processes using a malaria incidence dataset for Rwanda. We first compared the geographically weighted regression (WGR), the global random forest (GRF), and the geographically weighted random forest (GWRF) to examine the spatial non-stationarity in the non-linear relationships between malaria incidence and their risk factors. We used the Gaussian areal kriging model to disaggregate the malaria incidence at the local administrative cell level to understand the relationships at a fine scale since the model goodness of fit was not satisfactory to explain malaria incidence due to the limited number of sample values. Our results show that in terms of the coefficients of determination and prediction accuracy, the geographical random forest model performs better than the GWR and the global random forest model. The coefficients of determination of the geographically weighted regression (R2), the global RF (R2), and the GWRF (R2) were 4.74, 0.76, and 0.79, respectively. The GWRF algorithm achieves the best result and reveals that risk factors (rainfall, land surface temperature, elevation, and air temperature) have a strong non-linear relationship with the spatial distribution of malaria incidence rates, which could have implications for supporting local initiatives for malaria elimination in Rwanda.
Avdelning/ar
- Institutionen för naturgeografi och ekosystemvetenskap
- Centrum för geografiska informationssystem (GIS-centrum)
- Centrum för Mellanösternstudier (CMES)
- MECW: The Middle East in the Contemporary World
- BECC: Biodiversity and Ecosystem services in a Changing Climate
- LU profilområde: Naturbaserade framtidslösningar
Publiceringsår
2023-05-25
Språk
Engelska
Publikation/Tidskrift/Serie
Geospatial health
Volym
18
Issue
1
Dokumenttyp
Artikel i tidskrift
Förlag
University of Naples Federico II
Ämne
- Physical Geography
- Public Health, Global Health, Social Medicine and Epidemiology
Nyckelord
- variable importance
- partial dependent plot
- malaria incidence
- geographically weighted random forest
- spatial epidemiology
- Geographic information system (GIS)
- Artificial Intelligence (AI)
- Machine Learning (ML)
- Geospatial Artificial Intelligence (GeoAI)
Status
Published
ISBN/ISSN/Övrigt
- ISSN: 1970-7096