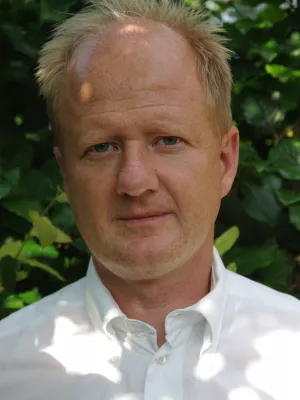
Lars Harrie
Professor
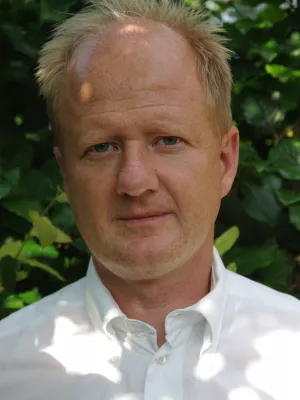
A feasibility study of applying generative deep learning models for map labeling
Author
Summary, in English
The automation of map labeling is an ongoing research challenge. Currently, the map labeling algorithms are based on rules defined by experts for optimizing the placement of the text labels on maps. In this paper, we investigate the feasibility of using well-labeled map samples as a source of knowledge for automating the labeling process. The basic idea is to train deep learning models, specifically the generative models CycleGAN and Pix2Pix, on a large number of map examples. Then, the trained models are used to predict good locations of the labels given unlabeled raster maps. We compare the results obtained by the deep learning models to manual map labeling and a state-of-the-art optimization-based labeling method. A quantitative evaluation is performed in terms of legibility, association and map readability as well as a visual evaluation performed by three professional cartographers. The evaluation indicates that the deep learning models are capable of finding appropriate positions for the labels, but that they, in this implementation, are not well suited for selecting the labels to show and to determine the size of the labels. The result provides valuable insights into the current capabilities of generative models for such task, while also identifying the key challenges that will shape future research directions.
Department/s
- Dept of Physical Geography and Ecosystem Science
- eSSENCE: The e-Science Collaboration
Publishing year
2024
Language
English
Publication/Series
Cartography and Geographic Information Science
Document type
Journal article
Publisher
American Congress on Surveying and Mapping
Topic
- Other Computer and Information Science
Keywords
- automated cartography
- deep learning
- generative adversarial networks
- image synthesis
- machine learning
- Map labeling
Status
Epub
ISBN/ISSN/Other
- ISSN: 1523-0406